Poker Bots
Background
Playing poker using bots is cheating. BTW, you must understand that if the business has profit, it cannot be easily forbidden. Using 'friendly' bots in poker rooms is a nature of poker boom supporting. Moreover, beating bots is not a problem for advanced players. BTW, there are a lot of newbies that cry about money lost in playing with 'bots'. What is Pokerbots? 6.176, or MIT Pokerbots, is a computerized poker tournament. Teams have one month to program a completely autonomous pokerbot to compete against other teams. Competitors must learn and apply concepts in economics, mathematics, and computer science not normally developed together in academic settings in order to conquer their opponents. Researchers say they have designed a bot called Pluribus capable of taking on poker professionals in the most popular form of poker and winning. Four-time World Poker Tour title holder Darren Elias.
Reinforcement Learning
Before I even begin though let's talk about what a poker bot even is. As mentioned, a poker bot is simply a non-human computer program that will play poker for you. Essentially you program the bot (or have somebody program it for you) to follow. Apr 20, 2020 A bot is essentially a piece of software that automatically plays online poker. The software analyses what’s happened at the table, then makes an automatic decision of what action to take.
Reinforcement Learning has grown in popularity in recent years, and since Google Deepmind's AlhpaGo emerged victorious against Lee Sedol and other GO Grandmasters, Reinforcement Learning has been proven to be an effective training method for neural networks, especially in cases of deterministic and non-deterministic gameplay. Libratus, a Poker playing Neural Network developed by Carnegie Mellon University, applies Reinforcement Learning techniques along with standard backpropagation and temporal delay techniques in order to win against Poker players across the world, including the winners of past Poker Grand Tournaments. However, Libratus does not use current deep learning and reinforcement learning techniques, as outlined by the AlphaGO or Deepmind papers. We wanted to explore the possible benefits of using Q-Learning to create a poker bot that automatically learns the best possible policy through self-play over a period of time.
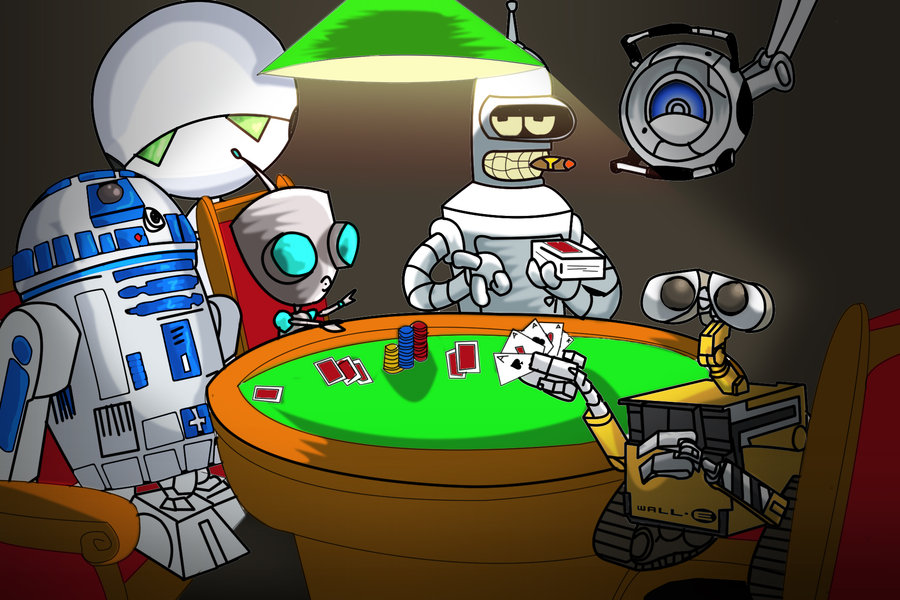
Q-learning
POKER BOT POKER BOT DOWNLOAD
Q-learning is the specific reinforcement learning technique we wanted to apply to our PokerBot. A complete explanation of Q-Learning can be found here. For our purposes, it will suffice to know that:
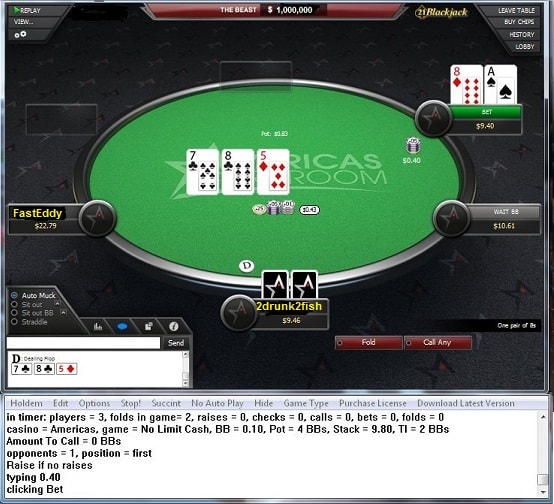
- Q-learning penalizes actions that may end up badly in the future
- Q-learning ALSO rewards actionst that may end up winning the game in the future
- We need action-state pairs: A list of all possible actions in all possible states
- A Q-function can then be generated
- This represents the BEST possible future reward if the action 'a' is taken in state 's'
This is the first part of Building a Poker Bot series where I describe my experience developing bot softwarefor online poker rooms. I’m building the bot with .NET framework and F# language which makes the task relativelyeasy and very enjoyable.
Screen recognition
For a human, the very first step to the ability to play poker is to understand the cards, what a hand is andwhat the value of your hand is. E.g. in Texas Holdem each player gets 2 hole cards which form a hand. Atthe showdown the player with the best hand wins.
Poker bots are no different, they also need to be taught the notion of cards and hands. A bot should “watch”the table and see which cards he is dealt with. There are several ways to achieve that but I go for a techniquecalled screen recognition, i.e. the bot makes a screenshot of a table and then reads the pixels to understandwhat’s going on. Very similar to what people do.
Image recognition in general is a tough task. Human beings are very good at interpreting vague images andrecognizing familiar objects. It’s much more difficult for computers. General image recognition (think showinga photo to your computer and asking whether there is an animal there) is very tough; corporations like Googleand Microsoft are spending numerous man-years and employ techniques like machine learning and neural networks.
Fortunately, poker table recognition is much easier. The images to be recognized are machine-generated, sothe same things are rendered more or less the same way all the time. It makes sense to keep the poker tablesize fixed to some predefined value which makes recognition task fairly easy.
Card recognition steps
There are 13 card faces (from Deuce to Ace) and 4 suits. All of them are just fixed-size images which we need to be able tomatch with. So we start with a screenshot of a poker table:
The table size is fixed, so are the left and the top pixel positions of hole cards. So, our first step is to extractthe small images of cards out of the big screenshot:
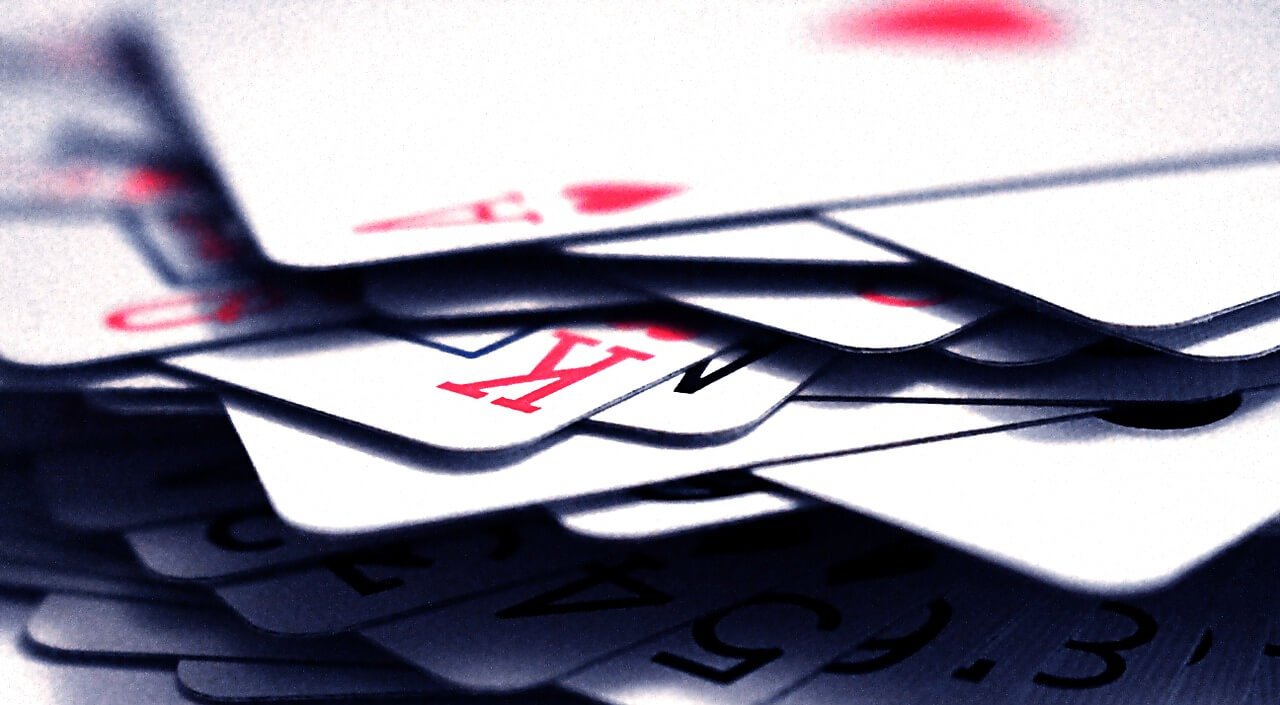
Now, we can take the recognition of card faces and suits separately. In our sample layout, suits are color coded.This is very friendly to humans and super simple for the bot. We pick the suit based on the color (ignoringthe white pixels):
This leaves us with the task of choosing between 13 card faces. The color information is not importanthere, we can make the image grey-scale. Moreover, we can reduce the color information to the single bit perpixel - call it white or black:
Now this mask is very simple, and we can compare it with 13 predefined masks for 13 cards pixel by pixel.The one with the biggest amount of matches wins.
Suit recognition
Let’s put some code at the table. We start with suit recognition. getSuit
function has typeColor -> string option
and converts the color of a pixel into the suit name, if possible. Hearts (“h”)are red, Diamonds (“d”) are blue, Clubs (“c”) are green and Spades (“s”) are black:
This function is used by getCardSuit
function of type (int -> int -> Color) -> int -> int -> string
.Its first argument is a function which returns the color of a pixel based on (x, y)
relative coordinates (starting with 0). The next two arguments are width and height of the cards. Result isthe same suit name that we described above. The function loops through all the pixels, gets a suit perpixel and then returns the suit which is the most popular among them. Alternatively, we could just returnthe first suit found, but my implementation looks more resilient:
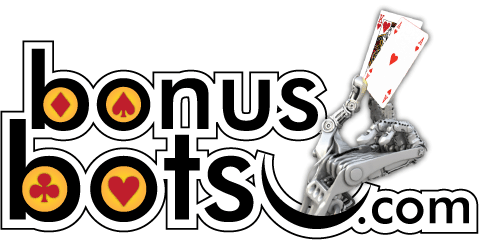
Producing the black & white pattern
getCardPattern
accepts the same parameters as getSuits
but returns seq<BW>
instead. This isa sequence of black or white pixels with a helper union type:
The function body enumerates the pixels and return black or white result as a flat sequence:
Card face recognition
Poker Bots: How To Beat Them!
Having a black and white pattern, we can compare it with the predefined patterns and pick themost similar one. A pattern is defined with a helper type
Pattern
is a sequence which is equivalent to the sequence we got on the previous step.Card
is a string of hand face value 2, 3, 4 .. A. getCardFace
has the typeCardPattern[] -> seq<BW> -> string
, it accepts an array of known patterns and a patternof the card to be recognized. It compares patterns pixel by pixel and returns the cardwhich has the biggest amount of matches:
Getting the known patterns
So how do we create an array of known patterns? It’s tedious to do manually, sowe use a bit of code generation.Basically we just take several screenshots of poker tables and feed them to the followinghelper function:
The function creates a string which can be copy-pasted into F# array of BW
.
Putting it all together
Here is the facade function that will be called from the outside:
The calling code looks like this:
leftX
, rightX
, top
, width
and height
are well-known parameters of cards locations within a screenshot,which are hard coded for a given table size.
Conclusion

The full code for card recognition can be found in my github repo. It’s just 75 lines of code which ismuch less that one could imagine for a task of image recognition. Similar code could be used to recognize otherfixed objects at poker table: dealer button location, action buttons, checkboxes etc. In the next part of thisseries I will show how to recognize non-fixed parts: text and numbers.
Proceed to Part 2 of Building a Poker Bot: String and Number Recognition.